The Common myths about Machine Learning
by Rebecca Harrison Project ManagerMachine learning is changing the dimensions of business in many industries. A report projects that the value added by machine learning systems shall reach up to $3.9 Trillion by 2022.
Machine learning is an exciting subject, and people are often confused between terms like machine learning, deep learning, and Artificial intelligence.
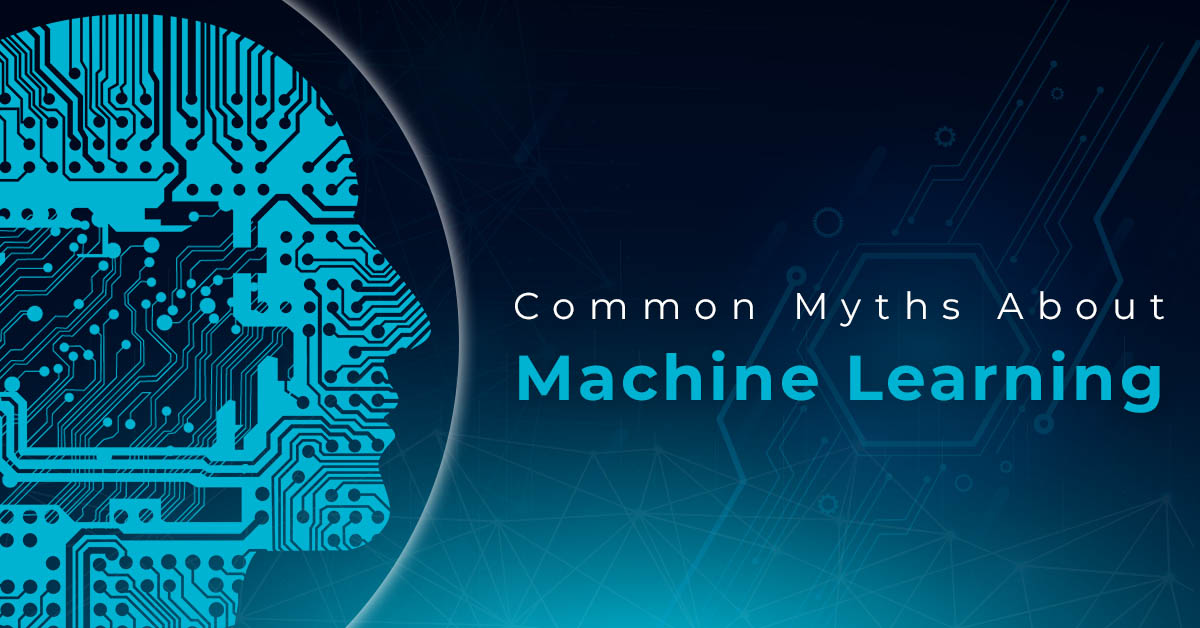
In this article, we shall try to dispel some myths regarding machine learning.
Difference between Machine Learning, Deep Learning, and Artificial Intelligence
▪️ Machine Learning:
Machine learning is a subset of AI. In machine learning, the programmers have written some algorithms that allow a system to make interferences from a given data and aid the system in making decisions.
Machine learning is essentially a technique in Artificial Intelligence. Machine learning requires human intervention.
▪️ Deep Learning:
Deep learning is a technique in machine learning, which loosely based on the patterns of how the human brain processes information. Deep learning is advancement in machine learning and is a subset of machine learning.
While machine learning requires some human interference, deep learning can work with minimal human intervention. Deep learning systems can literally "learn" on their own, making them as good as an artificial brain.
▪️ Artificial Intelligence:
Artificial intelligence is a technology that helps in building smart machines for handling complex tasks. Artificial Intelligence, on the whole, includes machine learning and deep learning.
Now that we have some basic understanding of machine learning, deep learning, and Artificial Intelligence, let’s have a look at some common myths associated with machine learning.
7 common misconceptions about machine learning (ML) technology
▪️ Machine learning systems will take our jobs
Many people have this strong belief that machine learning will create smarter robots that will gobble up their jobs. We forget that something similar happened during the onset of the industrial revolution, and the result was much different than what people feared.
There was a shift in the job market, and people upgraded themselves from laborious jobs to more knowledge-based ones. Machine learning is expected to have a similar impact by taking care of the repetitive tasks and letting us focus on the more critical tasks which require human expertise.
Machine learning is in no way designed to replace humans. Instead, it is designed to aid humans. Very few job roles are likely to be replaced, and the growth of machine learning has generated new job roles like the data analyst and the data scientist.
Thus the ML sector will give rise to a new breed of knowledge workers. Workers will feed data into the ML systems. They will also help in training the ML system to derive interference from this data.
Related Article: Why you should not be afraid of automation
▪️ Machine Learning systems can work independently without requiring humans
Another a common misconception is that machine learning systems do not require any human intervention and can work independently. Far from this, the ML systems are dependent on humans. Human programmers code the algorithms and make the necessary changes as and when they discover an anomaly. Also, humans will input data into the ML system from which the ML system derives interferences.
▪️ Machine learning systems cannot predict "Black swans."
Black swans are rare situations, like the Chernobyl nuclear disaster. Many people believe that it is not possible for the machine learning systems to accurately predict the occurrence of black swans. The thing is that they can.
A classic example of this would be the housing crash of 2008, where the machine learning systems accurately predicted the occurrence of a housing crash in the U.S. market while the traditionally programmed systems of banks could not predict the occurrence of this event.
▪️ It is sufficient to provide an ML system with vast chunks of data
In the world of computers, there is an adage, "garbage in, garbage out," this holds for machine learning systems also. You don't have to feed your machine learning system with gazillion bytes of data. Instead, you should focus on the quality of the data sets.
You will need to invest some time and resources to collect some good quality data by subjecting the data set to various tests. Your algorithm won't be able to deduce if the data which goes into the machine learning system is of poor quality.
▪️ Machine learning is concerned only with the analysis of data
Contrary to popular belief, ML systems are not just concerned with analyzing data. The the primary purpose of ML systems is to try and predict the future.
For instance, by analyzing your credit history, a Machine Learning system can predict whether you will be an excellent customer to lend.
▪️ Machine learning will produce robots with human-like intelligence
First of all, we need to understand that machines and humans are good at different things. While tools have been designed to perform calculations pretty fast, they cannot mimic human intelligence. Human intelligence is much more complicated than just chucking out some numbers.
For instance, while an ML system can predict that a baby will cry if not fed, it doesn't know what to do if food is not available. This is where natural human intelligence beats machine learning systems.
Humans can quickly decide what to do even if they haven't encountered a problem previously. While machine learning systems need to have data sets to identify and solve a problem.
While the advancements in the machine learning systems are encouraging these systems are still a far cry from being called as possessing human-like intelligence.
▪️ Machine learning cannot be used to establish causal relationships
It is a common myth that machine learning systems can be used only to find out co-relations between various data sets and cannot be utilized to establish causal relationships. Machine learning systems are used to experiment with different scenarios to find out a causal relationship.
For example,- e-commerce sites use ML systems with AI to experiment with various layouts, like putting the buy button in the center instead of the left corner. It helps them in identifying a design which can prove to be the best for improving sales. The systems on analyzing the website traffic and sales can accurately predict the effect of changing something in the layout.
For instance, the ML system can deduce that continually reminding people about their discarded carts will improve the conversion rate. The ML system is thus establishing a causal relationship between informing people about abandoned carts and improved sales figures.
Also Read: Accelerate Sales with Machine Learning and Artificial Intelligence
To wrap things up
Machine learning is proving it's worth in many industries like manufacturing, financial services, healthcare, and retail, to name a few. We hope that we have dispelled some of the myths associated with Machine Learning. It wouldn't be MLan incorrect to say that we have both overestimated and underestimated the potential of Machine learning systems.
Sponsor Ads
Created on Feb 11th 2020 05:10. Viewed 1,785 times.