How to Streamline Data Ingestion Using a Self-Service-Powered Approach
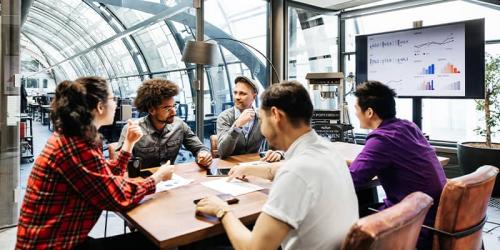
In the current times of disruption, companies are busy churning out actionable insights from complex, bi-directional data streams from customers or partners. That enables them to make proactive decisions and ultimately deliver value.
In case they fail, lots of issues can crop up:
Errornous analytic conclusions
Misleading reports
Inaccurate decision-making
For business users to easily consolidate data gathered from multiple sources and garner useful insights, data must be ingested in a digestible format first. So, data digestion is deemed extremely critical. In this blog post, we’ll explore data ingestion and its impact, challenges and how taking a self-service-powered approach can help companies overcome them.
Importance of Data Ingestion
As the name itself suggests, data ingestion involves the process of absorption of data that’s gathered from multiple sources into a particular database like a data lake or warehouse. Data analysts and integrators can access data residing in these places so that they can create integrations and use actionable intelligence to facilitate proactive decision-making.
As far as data sources are concerned, in-house apps, spreadsheets, databases, are the most common ones. It’s obvious that data collected from all these sources and more will stay in different formats, and so companies will need a robust data ingestion layer to ingest all this data - at speed and scale.
In other words, it’s evident that data ingestion solutions act as the backbone of any analytics architecture. The quality of insights extracted from various types of data is contingent on the way data is ingested.
The way data is ingested in a business ecosystem is often underpinned by different models or architectures. Essentially, it can be done in two different ways:
Batch-type ingestion: Batch-type ingestion is one of the most common techniques used by companies. In this type of ingestion, data is ingested in batches or groups. The source data is ingested periodically that is later transferred into a destination system. When the batches are made, data is ingested on the basis of a lot of ways: logical ordering, the activation of certain conditions, or a simple schedule. In scenarios where real-time processing is not required, this particular type of ingestion can be beneficial. It’s both simple and affordable.
Real-time ingestion: Also known as streaming or stream processing, real-time processing doesn’t involve groupings at all. In this type of ingestion, data is collected, transformed, and ultimately loaded as soon as it’s created or recognized by the layer. This specific type of ingestion is costly as businesses need systems to monitor sources to ingest information. It is preferably leveraged by users when real-time ingestion is required for optimized analytics.
Challenges of Data Ingestion
Just like any other process, data ingestion is also filled with challenges. The volume and variety of data are increasing each day. As a result of the increasing complexity, organizations find it challenging to ingest data. To add, data can come from myriad data sources, from transactional databases to other sources. With more data sources coming into the picture, data ingestion has become more tedious and time-consuming. IT integrators need to write long hours of code to ingest such large, complex data streams.
Another challenge is speed. It takes immense effort and time to develop and maintain data ingestion pipelines, especially when it comes to real-time processing. Organizations that still rely on legacy solutions struggle to ingest large streams of data on time. It takes a lot of IT’s time, and so they fail to focus on more high-value tasks.
Companies can take a self-service-powered approach to manage these challenges and help companies drive value at speed and scale.
How Self-Service Can Help
Self-service-based data ingestion solutions enable all business users (with minimal technical competency) to ingest large volumes of data into a data lake or data warehouse without delay. It simplifies big data ingestion in a way that it becomes more accurate and faster.
At the same time, IT can take up the role of governance and drive strategic tasks for innovation and growth.
As the data is ingested quickly, business users can leverage the ingested data to make valuable decisions. That enables companies to deliver delightful CXs and improve their ease of doing business.
Post Your Ad Here
Comments